This post has been republished via RSS; it originally appeared at: Microsoft Research.
This research paper was presented at the 2023 IEEE/CVF International Conference on Computer Vision (opens in new tab) (ICCV), a premier academic conference for computer vision.

When was the last time you were faced with a task you had no clue how to tackle? Maybe it was fixing a broken bike, replacing a printer toner, or making a cup of espresso? In such circumstances, your usual options might include reaching out to a knowledgeable friend or relative for assistance. Alternatively, you might resort to scouring the internet, conducting a web search, posing questions on online forums, or seeking out relevant instructional videos. But what if there were another option? What if you could turn to an AI assistant, or copilot, for help?
AI in the real world
Our daily lives are filled with a wide range of tasks, both for work and leisure, spanning the digital and physical realms. We often find ourselves in need of guidance to learn and carry out these tasks effectively. Recent advances in AI, particularly in the areas of large language and multimodal models, have given rise to intelligent digital agents. However, when it comes to the physical world, where we perform a significant number of our tasks, AI systems have historically faced greater challenges.
A longstanding aspiration within the AI community has been to develop an interactive AI assistant capable of perceiving, reasoning, and collaborating with people in the real world. Whether it’s scenarios like autonomous driving, robot navigation and manipulation, hazard detection in industrial settings, or support and guidance for mixed-reality tasks, progress in physical activities has been slower and more incremental compared with their fully digital counterparts.
Spotlight: Microsoft research newsletter
The promise and challenge of interactive AI “copilots”
There is great potential for developing interactive AI copilots to assist people with real-world tasks, but there are also obstacles. The key challenge is that current state-of-the-art AI assistants lack firsthand experience in the physical world. Consequently, they cannot perceive the state of the real world and actively intervene when necessary. This limitation stems from a lack of training on the specific data required for perception, reasoning, and modeling in such scenarios. In terms of AI development, there’s a saying that “data is king.” This challenge is no exception. To advance interactive AI agents for physical tasks, we must thoroughly understand the problem domain and establish a gold standard for copilots’ capabilities.
A new multimodal interactive dataset
As a first step in this direction, we are excited to share our paper, “HoloAssist: an Egocentric Human Interaction Dataset for Interactive AI Assistants in the Real World (opens in new tab),” presented at ICCV 2023 (opens in new tab). HoloAssist is a large-scale egocentric, or first-person, human interaction dataset, where two people collaboratively execute physical manipulation tasks. A task performer executes a task while wearing a mixed-reality headset that captures seven synchronized data streams, as shown in Figure 1. Simultaneously, a task instructor observes the performer’s first-person video feed in real time and offers verbal instruction.

HoloAssist contains a large collection of data, comprising 166 hours of recordings involving 222 diverse participants. These participants form 350 distinct instructor-performer pairs carrying out 20 object-centric manipulation tasks. Video 1 shows how tasks are recorded, while Figure 2 provides a task breakdown. The objects range from common electronic devices to rarer items found in factories and specialized labs. The tasks are generally quite demanding, often requiring instructor assistance for successful completion. To provide comprehensive insights, we’ve captured seven different raw sensor modalities: RGB, depth, head pose, 3D hand pose, eye gaze, audio, and IMU. These modalities help in understanding human intentions, estimating world states, predicting future actions, and more. inally, the eighth modality is an augmentation with third-person manual annotations, consisting of a text summary, intervention types, mistake annotations, and action segments, as illustrated in Figure 3.
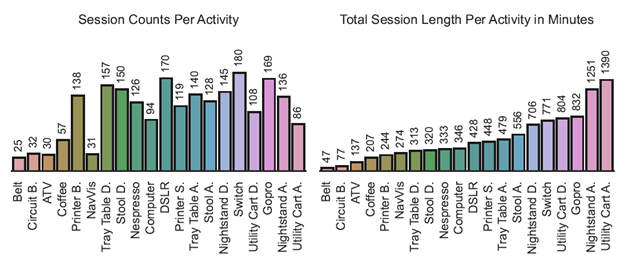
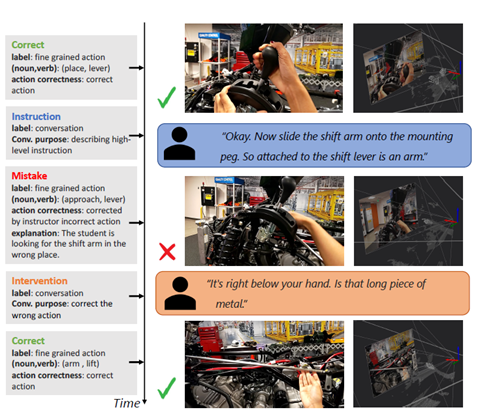
Towards proactive AI assistants
Our work builds on previous advancements in egocentric vision and embodied AI. Unlike earlier datasets, such as those listed in Table 1, HoloAssist stands out due to its multi-person, interactive task-execution setting. Human interaction during task execution provides a valuable resource for designing AI assistants that are anticipatory and proactive that can provide precisely timed instructions that are grounded in the environment, in contrast with current “chat-based” AI assistants that wait for you to ask a question. This unique scenario is ideal for developing assistive AI agents and complements existing datasets, which contribute rich knowledge and representation.

Finally, we evaluated the dataset’s performance on action classification and anticipation tasks, providing empirical results that shed light on the role of different modalities in various tasks. With this dataset, we introduce new tasks and benchmarks focused on mistake detection, intervention type prediction, and 3D hand pose forecasting, all crucial elements for developing intelligent assistants.
Looking forward
This work represents an initial step in broader research that explores how intelligent agents can collaborate with humans in real-world tasks. We’re excited to share this work and our dataset with the community and, anticipate numerous future directions, such as annotating object poses, investigating object-centric models of affordance and manipulations in AI assistance, and AI-assisted planning and state tracking, among others. We believe HoloAssist, along with its associated benchmarks and tools, will benefit future research endeavors focused on building powerful AI assistants for real-world everyday tasks. You can access the HoloAssist dataset and code on GitHub (opens in new tab).
Contributors
Taein Kwon, Mahdi Rad, Bowen Pan, Ishani Chakraborty, Sean Andrist, Dan Bohus, Ashley Feniello, Bugra Tekin, Felipe Vieira Frujeri, Marc Pollefeys
The post HoloAssist: A multimodal dataset for next-gen AI copilots for the physical world appeared first on Microsoft Research.